Ongoing technology development
Our discoveries have provided new insights into how experiences and memories are processed in the brain, but they have also revealed important limitations with our current approaches. Therefore, we are working to develop technology that enables more complex, longer-term and larger-scale investigations of neural activity.
Spyglass: a data analysis framework for reproducible and shareable neuroscience research
Sharing data and reproducing scientific results are essential for progress in neuroscience, but the community lacks the tools to do this easily for large datasets and results obtained from intricate, multi-step analysis procedures. To address this issue, we have worked with scientific computing experts at Lawrence Berkeley National Laboratory to create Spyglass, an open-source software framework designed to promote the shareability and reproducibility of data analysis in neuroscience. Spyglass integrates standardized formats (i.e., Neurodata Without Borders format) with reliable open-source tools, offering a comprehensive solution for managing neurophysiological and behavioral data. It provides well-defined and reproducible pipelines for analyzing electrophysiology data, including core functions like spike sorting. In addition, Spyglass simplifies collaboration by enabling the sharing of final and intermediate results across custom, complex, multi-step pipelines as well as web-based visualizations.
In parallel, we are working with Uri Eden, Mark Kramer and Adam Kepecs to develop new methods for quantifying information flow through neuronal circuits. It has become clear that information flow through the brain is dynamic: different areas and computations contribute to guiding behavior at different times, and our goal is to be able to estimate the moment-by-moment pattern of information flow across a distributed circuit using both point process and continuous state space models.
Very large-scale, multielectrode recording
While our recordings of neurons across multiple regions have been very informative, our current technology does not make it possible to record from sufficiently large ensembles of neurons in each area to understand, at a population level, how these areas interact and communicate. Simultaneous recordings of 50-100 hippocampal neurons makes it possible to decode both current position and the sequential “replay” seen during SWRs. Similarly, work from other labs has demonstrated that populations of ~100 neocortical neurons can be used to understand populations dynamics and to relate those dynamics to movement or other task variables. If we are to begin to understand computation in the distributed brain circuits that support memory formation, memory consolidation and memory-guided decision making we need to be able to understand how patterns of neural activity are related across regions. Furthermore, as the brain (and the memories stored in it) change over time, an ideal technology would permit very long term monitoring of populations of neurons across regions.
Those goals motivate our ongoing collaborations with colleagues at Lawrence Livermore National Laboratory, Lawrence Berkeley National Laboratory, Intan Technologies and SpikeGadgets. Working together we have developed a new set of flexible, biocompatible polymer electrodes, new electronics, new software and new surgical approaches that are making it possible to record from up to 1024 electrodes simultaneously, yielding high quality recordings from hundreds of neurons distributed across multiple brain regions in behaving animals. We have also been able to extend these recordings for many months, as well as to record continuously for 24 hours a day, 7 days a week.
We are continuing to refine that technology and to push toward higher density recordings with a longer-term goal of recording from thousands electrodes distributed across both cortical and subcortical structures. We hope to develop and apply technology to make it possible to record from entire brain circuits at single neuron spatial and millisecond temporal resolution to yield datasets that can be used to address a wide range of questions about how the brain learns, remembers, and decides.
Platform Overview Tracking individual single-units over time
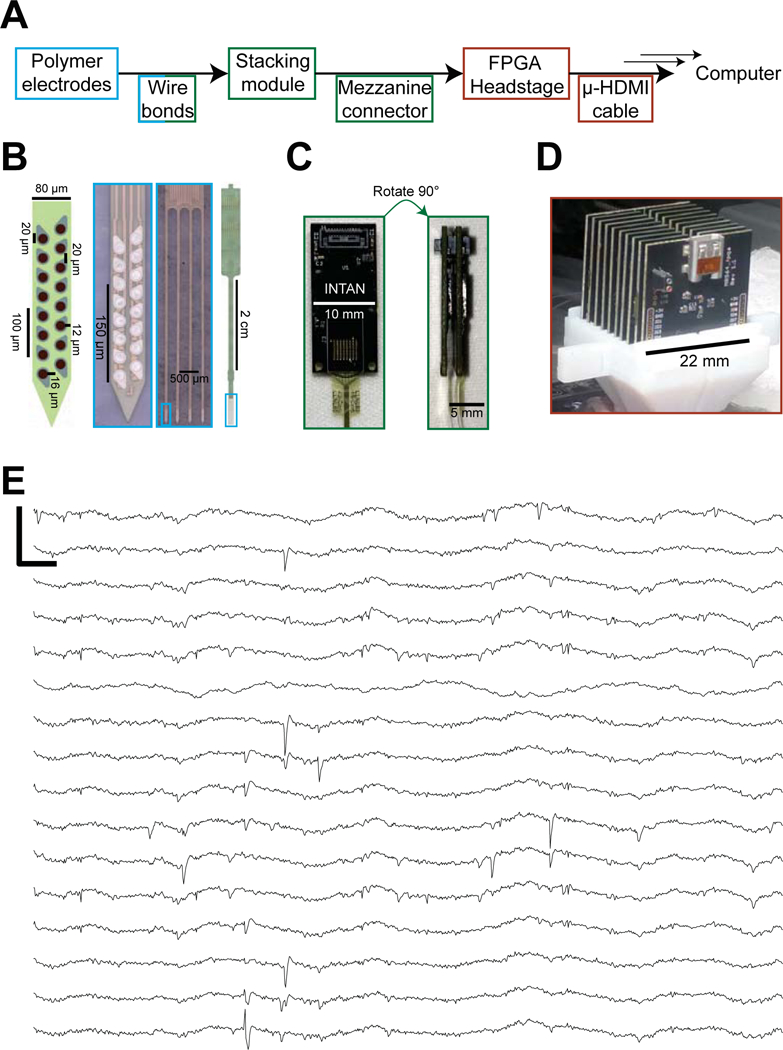
New algorithms for spike sorting
A rough calculation suggests that spike sorting of extended 1024 electrode datasets (wherein individual spike events are assigned to putative single neurons based on waveform characteristics) would require multiple person-years of time if we used standard manual or semi-automatic spike sorting approaches. We have therefore worked with Jeremy Magland, Alex Barnett, and Leslie Greengard of the Flatiron Institute to help develop, validate and optimize their new spike sorting algorithm and software, MountainSort (see also the forum). MountainSort allows for fully automated sorting of tetrode and polymer probe datasets, and runs approximately 10 times faster than real time, allowing for rapid sorting and, in the future, tracking of drift across long datasets.
Real-time, content-based feedback
Our very large scale recording approaches will help us identify the distributed patterns of neural activity that underlie memory processes, and we have already complemented these correlative observations with causal approaches where we demonstrated the importance of awake SWR events for learning and memory by interrupting all SWR events during learning. While that approach can be powerful, we also know that different SWR events can engage different sets of neurons with different representations. A fundamental goal of systems neuroscience is to understand how specific patterns of brain activity drive changes in downstream structures and behavior, and thus we need to be able to manipulate not only entire classes of events (e.g., SWRs) but also specific events based on their content. This will make it possible to assess how that content changes activity.
Making that possible requires reading out the content of brain activity as it occurs, and then detecting and manipulating patterns with specific content while leaving other patterns unaffected, all with roundtrip latencies from brain to computer to brain of at most a few milliseconds. Working in collaboration with Uri Eden of Boston University we are developing new clusterless decoding techniques (see Publications) and a new software and hardware infrastructure for real-time decoding and feedback. These tools should make it possible to establish the importance of specific patterns of brain activity in a way that was previously impossible.
Computational models
We are also developing computational models to help quantify and explain our animals’ behavioral responses. The goal of this effort is to derive sets of hidden state variables that can be then be related to observed patterns of neural activity. As our understanding of the relevant brain circuits and their dynamics progresses, we hope to be able to creating accurate models that explain how the hippocampus interacts with the cortex to support learning and memory processes.